AI is evolving at an incredible pace, but its growing energy demands pose a major challenge. Enter spintronic devices—new technology that mimics the brain’s efficiency by integrating memory and processing.
Scientists in Japan have now developed a groundbreaking spintronic device that allows for electrical control of magnetic states, drastically reducing power consumption. This breakthrough could revolutionize AI hardware by making chips far more energy-efficient, mirroring the way neural networks function.
Spintronic Devices: A Game-Changer for AI Hardware
AI is rapidly transforming industries, but as these technologies evolve, so does their demand for power. To sustain further advancements, AI chips must become more energy efficient.
This is where spintronic devices come in. By integrating memory and computing functions—similar to how the human brain operates—they offer a promising foundation for low-power AI chips.
Now, researchers from Tohoku University, the National Institute for Materials Science, and the Japan Atomic Energy Agency have developed a groundbreaking spintronic device. This new technology enables the electrical mutual control of non-collinear antiferromagnets and ferromagnets, allowing for efficient switching of magnetic states. In practical terms, it can store and process information using significantly less energy, much like a brain-inspired AI chip.
This breakthrough could pave the way for a new generation of AI hardware that is both highly efficient and energy-saving. The findings were published in Nature Communications on February 5, 2025.
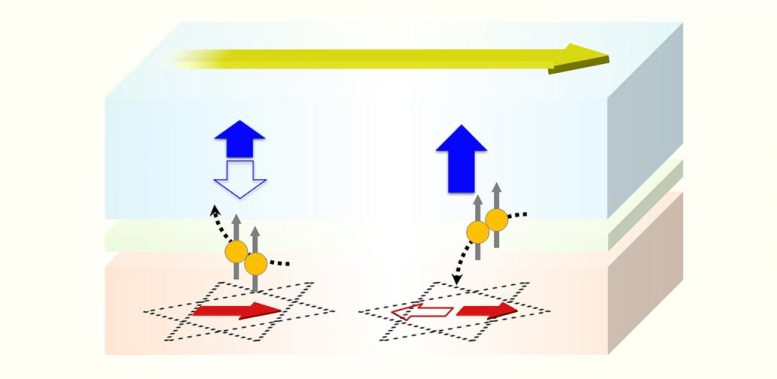
Revolutionizing AI with Multi-State Magnetic Control
“While spintronic research has made significant strides in controlling magnetic order electrically, most existing spintronic devices separate the role of the magnetic material to be controlled and the material providing the driving force,” says Tohoku University’s Shunsuke Fukami, who supervised the research.
These devices have a fixed operation scheme once fabricated, typically switching information from “0” to “1” in a binary fashion. However, the new research team’s breakthrough offers a major innovation in electrically programmable switching of multiple magnetic states.
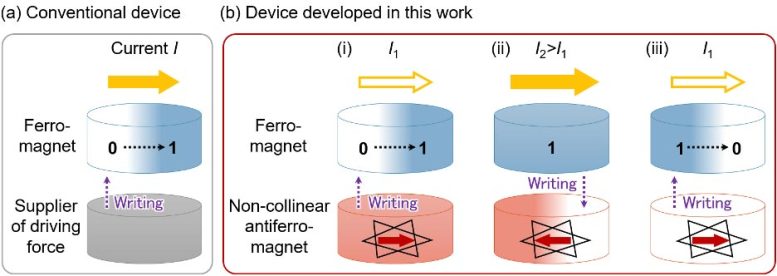
Harnessing the Power of the Magnetic Spin Hall Effect
Fukami and his colleagues employed the non-collinear antiferromagnet Mn3Sn as the core magnetic material. By applying an electrical current, Mn3Sn generates a spin current that drives the switching of a neighboring ferromagnet, CoFeB, through a process known as the magnetic spin Hall effect. Not only does the ferromagnet respond to the spin-polarized current, but it also influences the magnetic state of Mn3Sn, enabling the electrical mutual switching between the two materials.
In their proof-of-concept experiment, the team demonstrated that information written to the ferromagnet can be electrically controlled via the magnetic state of Mn3Sn. By adjusting the set current, they were able to switch the magnetization of CoFeB in different traces representing multiple states. This analog switching mechanism, where the polarity of the current can change the sign of the information written, is a key operation in neural networks, mimicking the way synaptic weights (analog values) function in AI processing.
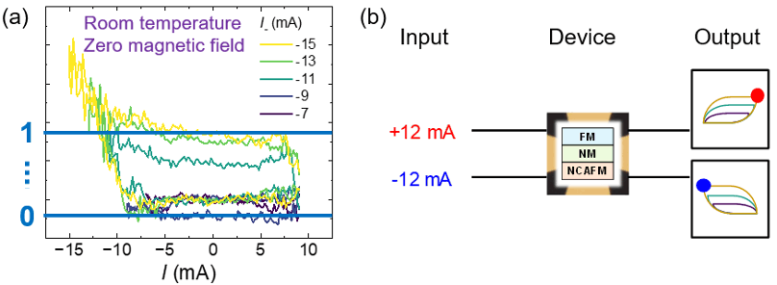
Paving the Way for Energy-Efficient AI Chips
“This discovery represents an important step toward the development of more energy-efficient AI chips. By realizing the electrical mutual switching between a non-collinear antiferromagnet and a ferromagnet, we have opened new possibilities for current-programmable neural networks,” said Fukami. “We are now focusing on further reducing operating currents and increasing readout signals, which will be crucial for practical applications in AI chips.”
The team’s research opens new pathways for improving the energy efficiency of AI chips and minimizing their environmental impacts.
Reference: “Electrical mutual switching in a noncollinear-antiferromagnetic–ferromagnetic heterostructure” by Ju-Young Yoon, Yutaro Takeuchi, Ryota Takechi, Jiahao Han, Tomohiro Uchimura, Yuta Yamane, Shun Kanai, Jun’ichi Ieda, Hideo Ohno and Shunsuke Fukami, 5 February 2025, Nature Communications.
DOI: 10.1038/s41467-025-56157-6

News
Reawakening Dormant Nerve Cells: Groundbreaking Neurotechnology Restores Motor Function
A new electrical stimulation therapy for spinal muscle atrophy (SMA) has shown promise in reactivating motor neurons and improving movement. In a pilot clinical trial, three patients who received spinal cord stimulation for one [...]
AI’s Energy Crisis Solved? A Revolutionary Magnetic Chip Could Change Everything
AI is evolving at an incredible pace, but its growing energy demands pose a major challenge. Enter spintronic devices—new technology that mimics the brain’s efficiency by integrating memory and processing. Scientists in Japan have [...]
Nanotechnology for oil spill response and cleanup in coastal regions
(Nanowerk News) Cleaning up after a major oil spill is a long, expensive process, and the damage to a coastal region’s ecosystem can be significant. This is especially true for the world’s Arctic region, [...]
The Role of Nanotechnology in Space Exploration
Nanotechnology, which involves working with materials at the atomic or molecular level, is becoming increasingly important in space exploration. By improving strength, thermal stability, electrical conductivity, and radiation resistance, nanotechnology is helping create lighter, more [...]
New Study Challenges Beliefs About CBD in Pregnancy, Reveals Unexpected Risks
CBD is gaining popularity as a remedy for pregnancy symptoms like nausea and anxiety, but new research suggests it may not be as safe as many believe. A study from McMaster University found that [...]
Does COVID increase the risk of Alzheimer’s disease?
Scientists discover that even mild COVID-19 can alter brain proteins linked to Alzheimer’s disease, potentially increasing dementia risk—raising urgent public health concerns. A recent study published in the journal Nature Medicine investigated whether both mild and [...]
New MRI Study Reveals How Cannabis Alters Brain Activity and Weakens Memory
A massive new study sheds light on how cannabis affects the brain, particularly during cognitive tasks. Researchers analyzed over 1,000 young adults and found that both heavy lifetime use and recent cannabis consumption significantly reduced brain [...]
How to Assess Nanotoxicity: Key Methods and Protocols
With their high surface area and enhanced physicochemical properties, nanomaterials play a critical role in drug delivery, consumer products, and environmental technologies. However, their nanoscale dimensions enable interactions with cellular components in complex and [...]
Nanotech drug delivery shows lasting benefits, reducing need for repeat surgeries
A nanotechnology-based drug delivery system developed at UVA Health to save patients from repeated surgeries has proved to have unexpectedly long-lasting benefits in lab tests – a promising sign for its potential to help human patients. [...]
Scientists Just Found DNA’s Building Blocks in Asteroid Bennu – Could This Explain Life’s Origins?
Japanese scientists detected all five nucleobases — building blocks of DNA and RNA — in samples returned from asteroid Bennu by NASA’s OSIRIS-REx mission. NASA’s OSIRIS-REx mission brought back 121.6 grams of asteroid Bennu, unveiling nitrogen-rich organic matter, including DNA’s essential [...]
AI-Designed Proteins – Unlike Any Found in Nature – Revolutionize Snakebite Treatment
Scientists have pioneered a groundbreaking method to combat snake venom using newly designed proteins, offering hope for more effective, accessible, and affordable antivenom solutions. By utilizing advanced computational techniques and deep learning, this innovative [...]
New nanosystem offers hope for improved diagnosis and treatment of tongue cancer
A pioneering study has unveiled the Au-HN-1 nanosystem, a cutting-edge approach that promises to transform the diagnosis and treatment of tongue squamous cell carcinoma (TSCC). By harnessing gold nanoparticles coupled with the HN-1 peptide, [...]
Global Trust in Science Is Stronger Than Expected – What’s Next?
A landmark global survey conducted across 68 countries has found that public trust in scientists remains robust, with significant support for their active involvement in societal and political matters. The study highlights the public’s [...]
Microplastics in the bloodstream may pose hidden risks to brain health
In a recent study published in the journal Science Advances, researchers investigated the impact of microplastics on blood flow and neurobehavioral functions in mice. Using advanced imaging techniques, they observed that microplastics obstruct cerebral blood [...]
AI Surveillance: New Study Exposes Hidden Risks to Your Privacy
A new mathematical model enhances the evaluation of AI identification risks, offering a scalable solution to balance technological benefits with privacy protection. AI tools are increasingly used to track and monitor people both online [...]
Permafrost Thaw: Unleashing Ancient Pathogens and Greenhouse Gases
Permafrost is a fascinating yet alarming natural phenomenon. It refers to ground that remains frozen for at least two consecutive years. Mostly found in polar regions like Siberia, Alaska, and Canada, permafrost plays a [...]